The AI Arms Race
Navigating the Future of Marketing and Technology
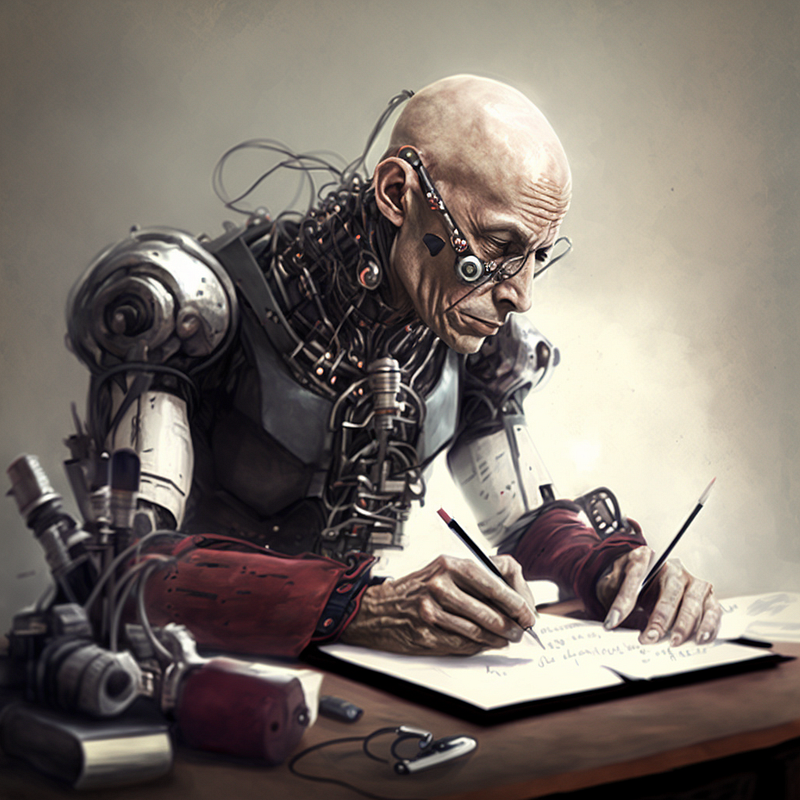

Introduction
In the rapidly evolving world of technology, AI has taken center stage, transforming industries at an unprecedented pace. Even early adopters, who pride themselves on staying ahead, may struggle to keep up with the AI arms race. As an entrepreneur with a decade of growth hacking experience and two GPT-4 powered apps under my belt, I’ve observed this swift progress and noticed that many early adopters are actually lagging behind. To successfully navigate the future of marketing and technology, it’s crucial to grasp AI’s implications for your situation. Here are some insights to help you stay ahead in this dynamic landscape.
Understanding AI Development
The AI Hype Cycle is unique in history. Coming on the heels of Covid, stonks, crypto shills, and every other Current Thing, it is easy to dismiss AI as just another thing the discourse wants you to follow. I’ve met many early adopters, in particular, who tried a tool like Jasper.AI or Copy.AI, quickly saw the limits of what they offered, and concluded they could write this off for a while.
Others who have worked in AI longer than OpenAI have expressed general skepticism. If you sold anything with the name “AI” around 2016, you might be inclined to see that this is all basic Machine Learning without a premium mediocre name to raise the price tag.
Both of these positions are mistaken. LLMs (Large Language Models) are a form of AI producing something revolutionary, and it’s mostly happened just in the last six months.
Deciphering the AI Version Numbers
Part of the blindspot here is in the simple version numbering. Consider the versioning for iPhones: at this point, most Americans decline to buy every version, treating each new edition as basically a minor improvement. Although they continue to make impressive gains in battery life and video quality, there simply are few wow moments left in the iPhone development cycle, and you can barely tell the difference between a 12 and a 14.
If iPhone numbering is linear, AI numbering is more like the Richter Scale for earthquakes: every version represents an order of magnitude improvement in quality. Consider the documented improvements in GPT-4’s ability to pass standardized tests:
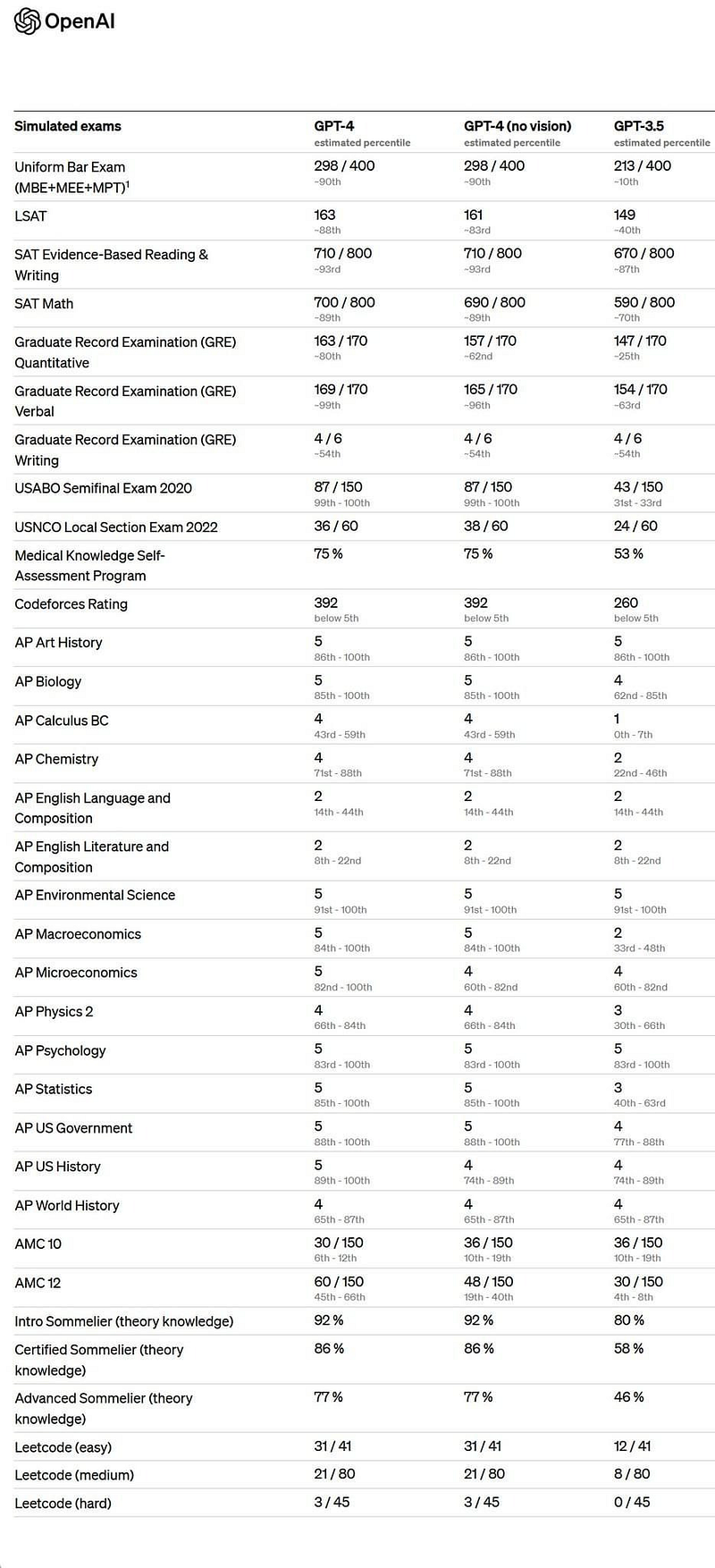
Unlike hardware development, LLMs don’t really have a roadmap — there isn’t a planned list of features to add in 2025. Instead, we’re discovering in real time as models tuned with a higher number of parameters develop skills in some areas and not in others. For a company producing these models, they can only focus on reducing the parameters, keeping development costs low and training time high.
Your Affordable McKinsey Consultant
Another common blindspot I’ve seen is a misunderstanding of the problems LLMs can solve. Typically a user expects GPT to get the right answer to a question, and when it makes a mistake, they write it off as useless.
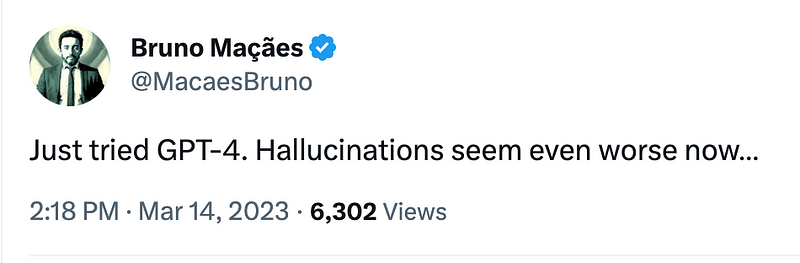
It’s more helpful to treat GPT not as a diligent source of truth, but as a very affordable college educated McKinsey consultant. Like consultants, GPT takes in a broad amount of information and produces plausible sounding bullshit which is often useful. Also like consultants, GPT is unable to say “I don’t know.” It believes its own bullshit, mostly.
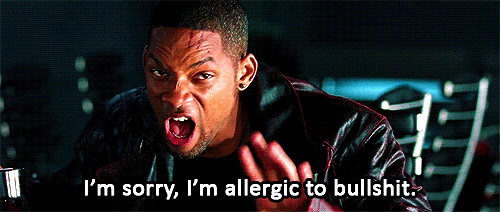
Rejecting the value of a McKinsey consultant is missing the point: you can now have an effectively unlimited army of McKinsey consultants for pennies on the dollar, producing bullshit 100x faster, without the personal ambition to replace your CEO.
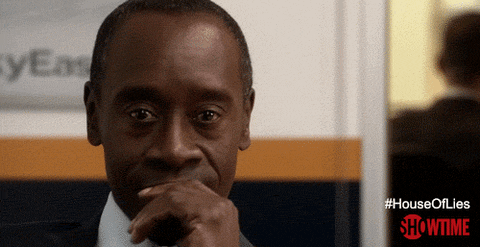
We don’t yet know what an army of apolitical, coachable, and blissfully unambitious McKinsey consultants can achieve — before GPT, this breed did not exist!
Complicated Problems and Cynefin: Navigating the Problem Space
As we learn to make sense of what problems an LLM can solve, I’ve found it helpful to consider the Cynefin framework from Dave Snowden.
The Cynefin framework is a decision-making tool that helps organizations identify the nature of problems they face and determine the best approach to address them. The framework primarily classifies problems into four domains, simple, complicated, complex, and chaotic.
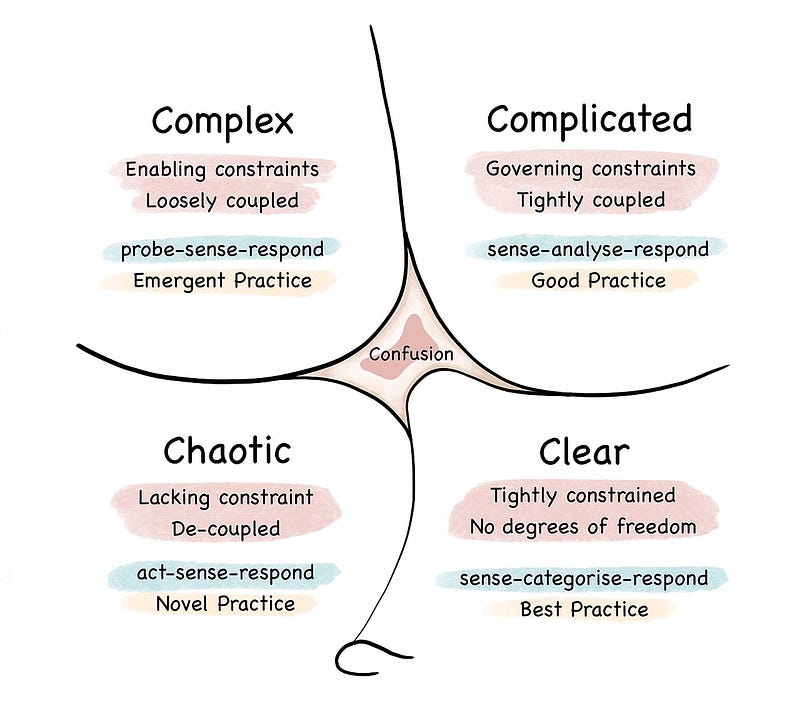
LLMs fit squarely in the complicated domain. Complicated problems are the problems that require expertise and specialized knowledge to solve. They often have multiple correct answers, and you can arrive at these answers by taking a snapshot of the problem, analyzing it, and applying good practices. In this domain, LLMs excel because they have vast knowledge, they can provide multiple solutions to a problem, and the answers are rarely as black and white, providing more room for error.
LLMs can fail at simple problems and complex problems.
Simple problems are those that can be defined by an SOP. They follow strict decision trees to get to one specific answer. Actually LLMs can do very well here, but you have to set their temperature close to 0.0 (the level of GitHub CoPilot) rather than 0.7 (the estimated level of ChatGPT.)
Complex problems are those that change more dynamically: examples include sales, dance, firefighting, aerial dogfighting, and improv theater. In these domains, humans have learned to move fast by relying on heuristics rather than analysis, and learning to adjust course as they go. LLMs struggle here, today, because they cannot sense what happens after we take their advice.
In the realm of marketing and technology, understanding the Cynefin framework and how LLMs succeed within it can help you leverage AI more effectively. By recognizing the limitations of LLMs, and adjusting your questions to account for these limitations, you can choose the right tool for each situation without rejecting the technology itself.
Implications for Marketing
As we navigate the complex landscape of AI-driven disruption, marketers, in particular, face a unique set of challenges. Not only do they confront the direct risks posed to their profession, but they must also grapple with the potential loss of entire buyer segments and the erosion of once-reliable marketing channels. However, amidst these challenges lie opportunities for marketers willing to reexamine what their profession requires. Embracing this new reality and adapting to the changes it brings will be key to thriving in the age of AI.
Challenge: Rapid Deflationary Cycles and Digital Goods
In the 1990s, technology incumbents often loudly announced “vaporware”, coming soon releases that promised enough features to discourage their customers from switching to the new new thing.
LLMs risk doing something similar: with such a rapid pace of innovation, most buyers will be discouraged from committing to more than a monthly contract, knowing that next week there could be another 10x improvement announced by the competition.
I’m not immune: this week I decided to upgrade an AI product that I enjoy, and when I went to the pricing page, I quickly changed the pricing to monthly. This product is easily a market leader, but we’re in uncharted territory, where a market leader might fall to #5 all at once. Signing for an annual contract would financially commit me to a potential losing technology; worse, it might lead to blinders on what else is out there.
As enterprise buyers discover this pace of change, I expect to see similar shifts in buying behavior. Before LLMs, a software vendor mostly competed with other vendors, the busy in-house team, and the option to do nothing. With LLMs, you now compete with other vendors that can move 10x faster, an in-house team that may have just discovered LLMs, and the fear that your price might prove to be a liability in just a few months.
To win customers in this environment, you need to demonstrate that you will keep up with the arms race, or offer AI for free while selling a less volatile product or service.
Since the emergence of the SaaS business model and the era of low interest rate VC money, we’ve seen a priority placed on recurring revenue for software. This may rapidly shift, as the competition for this market just became bloodier.
Challenge: The Death of SEO
SEO is older than most digital marketers, so it may come as a surprise that its days are numbered. Here, too, there are threats from multiple directions.
The first threat is the impact of LLMs on content production. I know, dear marketer, you’ve survived link farms, dictionary rewrites, and a generation of VAs producing endless garbage content. We even survived 2016 and the Moldovan fake news generators. But this time is different: LLMs don’t just produce cheaper content, they produce better content, faster. Cheaper than a VA, faster than a journalist, better than a bestselling author. This tsunami of content means that under normal circumstances you would need to 10x your game just to keep up and win the SERPs.
The trouble is, there may not be any SERPs left to win. When Bing announced their chat bot, Sydney, it set off an accelerated race in the search engine category. DuckDuckGo and Yahoo, both using Bing to power their search indexing, quickly moved to create their own chat bots using the same data. Google, which has slowly replaced SERPs with AI generated content for years, now has no reason to maintain their slow pace.
In a year, it’s likely that 90% of search results will end in an answer from a chat bot. Google and Bing will continue to index your content, and use it to answer questions, but you will no longer receive the traffic for your effort.
Some reading this will insist that they’ve survived algo updates before, and Google can simply signal boost content that was created by humans or before GPT existed. Indeed, if Google knew what was written by a human, they might be able to offer an exclusive search engine that returns human generated writing. But Google cannot recognize content generated by an LLM. While some LLMs claim to recognize their own signature and identify their own writing, there is no universal text watermarking technology that enables content to be traced back to any AI source. Were this to exist, you could simply check your content against the model, and then launder your content through other LLMs until it comes out clean.
Google never liked SEO; they will continue as a business far longer than SEO. Until we identify a tactic to inject your brand and URL into AI responses, the upside for SEO will continue to dwindle. Its days are numbered.
Who wins in Marketing?
As the AI revolution disrupts the SEO and content marketing landscape, there remain viable strategies to succeed in this new world. Although it is still early innings, three key strategies emerge as potential winners.
Opportunity: Conspicuous Human Connection
In a world saturated with AI-generated content, the conspicuously human will be seen as more valuable. Word of mouth marketing, referrals, personal stories, community and IRL events will stand out.
Of these, community and events are the only ones favorable to new companies, so lets discuss these a bit. Most of the innovation in LLMs will escape the attention of community and event organizers, who will default to doing the same thing they’ve always done. Yet there is significant advantage to leverage LLMs in these channels:
- Dump everything you know about your community members into GPT, and you can synthesize the overall vibes they will best respond to
- Use AI to organize events, and you can likely triple the events on the same labor budget
- Use AI to scan your community for inside jokes and suggest personalized schwag you can send to members
These indirect applications of AI will succeed because they will be less obvious to your competition, and therefore less easily copied.
Opportunity: Engineering as Marketing
The best traction channel you’ve never tried is about to have its moment.
I’ve been a long time proponent of producing software artifacts to generate leads and pipeline, but despite strong case studies on its success, most companies haven’t tried it. Tech companies, in particular, are loath to put a valuable software engineer in the marketing department when they could be working on the core product. Non-tech companies generally can’t afford software engineers and don’t know what to do with them.
So this channel has gone mostly dormant, full of opportunity, marketers, and their good ideas that would be created but for the lack of programming skills.
Until now.
In January I created a web app in less than 24 hours, using APIs I had never tried before. This past Sunday, I created a chrome extension in an hour to query your browser and download all web history.
These are the benchmarks of a 10x programmer, but I am not a 10x programmer: I am a marketer with GPT-4.
Now, marketers have the tools available to produce lead magnets that look like web apps. The Hubspot Website Grader, which generated over $5M in pipeline, can be reproduced in a few hours. Want an ROI calculator? This is probably a half day of work. Add 15 minutes if you want GPT to change the colors and font to match your brand.
While the cost for marketers to generate software decreases, the upside actually increases. As covered earlier, buyers hesitate to pay directly for software that might be obsolete in a month. This lowers the total market for SaaS, but it increases the total market for free software. Buyers will gladly try a new web app that moves them closer to a solution, if it buys them time to identify the winners in the market before investing real money.
Further, web apps are a potential winner in a world where SEO dies. While a chat bot can aggregate data from your content marketing efforts and replace you, it cannot (yet) use your web app to answer a question for a user. This means that effective, high ranking web apps will have a shot at getting endorsed by the chat bots, along with a link so the users can try them out.
In short, marketers with GPT-4 can now produce web apps faster than engineers can, in an environment where free web apps will likely escape the chatbot summarization layer.
Opportunity: Privileged Data
As more marketers discover the leverage in producing their own software, the enduring value will shift from free toy web apps to apps that attract and leverage unique data.
To understand this, consider what Google can and cannot summarize for you with a chat bot:
- Where to eat: Google wins
- Where to sleep: Google wins
- Who to sleep with: Google loses
Websites that (reluctantly) ceded their data to search engines, such as Yelp, Tripadvisor, and ESPN, will mostly lose out to chat bots. If Google hesitated to replace them before, with Antitrust litigation lurking just around the corner, they now have the plausible threat of Bing to justify throwing these companies out for the sake of survival.
Meanwhile, the companies that hold proprietary data will likely thrive awhile longer: Tinder, most social media apps, and most mobile apps cannot be disrupted by a Google chat bot absent some admission of aggressive scraping practices. Which is unfortunate, because I for one would trust Google to recommend a date more than I trust Tinder. But I digress.
As a marketer, you’ll need to seek out methods to aggregate specific data and knowledge that cannot be easily found in Google or Bing. With this knowledge, you’ll be able to generate your own chat bot fiefdom.
The Future of AI and Marketing: Navigating Uncharted Waters
The craziest thing about AI, today, is that it will never perform worse than it performs now.
The pace of change is truly astounding, causing makers and buyers alike to hesitate in their next move. Like GPT-4, we don’t actually know what comes next, so the best we can do is put one word up after the next until we hit on something worthwhile.
That said, there are some clear signs on the map of the future that are worth planning for.
Two Potential Killer Apps
There are two incredible apps emerging that actually exceed anything in Black Mirror.
Just in Time Software: Lindy
Lindy is a startup that proposes to ingest all of your information and write just in time software to do anything you want. Get a list of your Google Contacts? Just ask Lindy and it will write the program to do it. Import these into your Hubspot? Just ask Lindy.
I’m wary that Lindy has no published privacy controls, and they cannot QA the quality of the software written. This is truly a religious choice to believe that AI is ready to write software to such a high standard that it need never be tested.
Still, most people seem to want GPT to be an oracle, and Lindy fits this worldview well. I’m bearish on the product, but bullish on the company and the category itself.
Better than Black Mirror: Rewind.AI
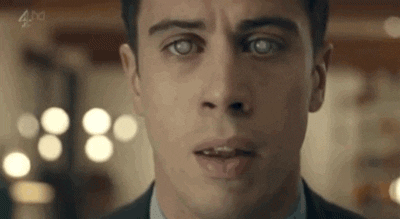
One of the early episodes of Black Mirror suggested a future where we all had implants to record everything in our lives, available to rewind and search.
Rewind.AI may actually do more. Founded by Dan Siroker, Rewind offers a new way to record everything in your (macbook-connected) life to make it easily searchable. As of January they approximated the same upside of that Black Mirror episode. But then GPT-4 arrived, and Rewind now proposes to send enough information from your data stash to let GPT-4 answer questions about… anything in your life.
While everyone else focuses on trying to make the best AI, Rewind has cleverly focused on the thing every AI requires: secure, personal, unique data.
Look for Apple to acquire them and integrate into their OS.
GPT-4 gets a memory
GPT-3 (what you’ve probably used in ChatGPT) is able to handle about 3000 words in its contextual memory about your question. The API named each call a “prompt” because that is how it worked: as a zero shot or one shot prompt.
GPT-4, today, handles 6000 words. Later this year, they will release a version that handles 24,000 words, about ⅓ of the median book. This alone will lead to another step change in innovation, particularly in pattern matching and real-time analysis.
At this rate, the number of words that can be loaded doubles every 3–6 months. In a year, that places the memory available at 96,000 words. This alone will create upside we cannot even imagine.
The Dawn of OpenAI Plugins
Until recently, the LLMs were limited to read-only access of their existing knowledge. Most observers assumed OpenAI would eventually offer plugins and become a platform, but few imagined it would happen overnight.
So naturally it happened overnight.
We can now see that the near future includes plugins which allow an LLM to not just synthesize ideas from its existing knowledge, but go out to the internet and APIs to interact with the world.
I’m actually bearish on this, because OpenAI is trying to tread carefully, wary that the Singularity could show up at any moment, and I haven’t yet seen a killer app (though Patio11 would suggest Zapier is the clear killer app.)
The Emergence of Free and Decentralized AI Models
With many people concerned about censorship, and the prevailing decentralization ethos of crypto still in the air, there are a lot of free models getting released and optimized.
Here we can look to trends in image generation for guidance. While centralized models showed promise, it was only with the release of stable diffusion as a free tool that the innovation took off in the space.
Similarly, look for advances in the LLM space with LLaMa and others. Its actually possible that OpenAI will be disrupted by a free, open-source, downloaded model that works 90% as well for the cost of your laptop speed.
This scenario particularly benefits Apple, as they’ve already optimized their chips to handle AI better than other laptops, and they lack any viable horse in the centralized AI race.
The Giants Lurking in the Shadows
With so much innovation, its easy to miss that we’ve seen very little from FAANG.
Meta leaked LLaMa, and it holds promise, but they have no direct commercial interest in it.
Google is scrambling. Sergey and Larry are back, making pull requests, and hoping they can release enough meaningful product to survive. If Google Bard is any indication, they will be limping along for awhile.
Apple too is scrambling, albeit more quietly. Siri has never led in anything, and Apple is at risk to lose the AI space much as they lost social media.
Expect both to make major announcements in the next few months as a means to take back the media narrative.
Conclusion
I asked ChatGPT to give me feedback as the editor of a major business magazine, but it refused to accept this much text. Undaunted, I went to the GPT-4 API and was able to get constructive feedback to make the entire post better.
The limits of these tools are mostly unknown and malleable: generally, your imagination will be the bottleneck.
I asked GPT-4 to write a conclusion but it was rather boring. Instead, here is the conclusion as a limerick:
In the world of AI, we’re racing,
Marketers’ challenges, they’re facing.
Stay ahead of the curve,
Adapt and observe,
In this landscape, there’s no time for wasting.